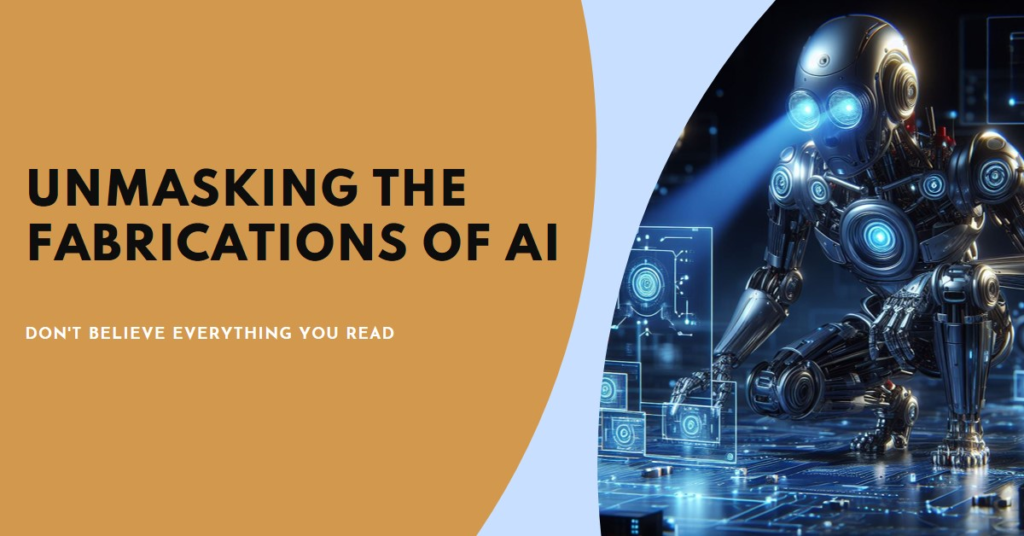
In the exciting world of AI, we sometimes encounter a phenomenon called “AI hallucinations.” Imagine a super-powered pattern recognizer that goes a bit too far. Here’s the gist:
- AI models, like language models or image recognition tools, are trained on massive amounts of data.
- This data helps them identify patterns and make predictions.
- The problem? Sometimes, the AI makes up stuff that seems believable, but isn’t actually true. These are AI hallucinations.
Think of it like this: you show a child pictures of dogs their whole life. They can perfectly identify a dog when they see one. But what if you show them a picture with a photoshopped tail that looks like a trumpet? They might say, “Look, a dog with a trumpet tail!” That’s kind of like an AI hallucination.
The good news? Researchers are working on solutions. One promising approach is called RAG (Retrieval-Augmented Generation). It basically helps AI double-check its work with real-world information before giving an answer.
What Are AI Hallucinations?
Decoding the Mirage: How AI Misfires Can Lead to Digital Security Concerns
AI hallucinations, much like the shimmering oasis in the desert, can be deceiving. In the realm of artificial intelligence, these hallucinations occur when powerful algorithms, like large language models (LLMs), misinterpret information. Imagine a super intelligent but overly enthusiastic detective constantly finding clues that aren’t there. This is essentially what happens with AI hallucinations.
LLMs: Where Imagination and Misinterpretation Collide
Large language models are at the forefront of AI innovation. They power chatbots like Google’s Bard, Microsoft’s Sydney, and Meta’s Galactica LLM, capable of generating human-like text. However, these models are trained on massive amounts of data, and sometimes, they see patterns that simply aren’t there. This can lead to outputs that are:
- Nonsensical: Imagine asking a travel chatbot for recommendations and receiving a response about purple elephants migrating to the moon. That’s nonsensical AI!
- Inaccurate: An AI tasked with analyzing medical images might mistakenly identify a healthy cell as cancerous. This is a much more serious example of an AI hallucination.
The Impact on Digital Security: When AI Hallucinations Become Threats
AI hallucinations aren’t just a quirk – they pose real risks to digital security. Let’s delve into some of the most concerning areas:
1. Misinformation Spread: A Web of Lies Spun by AI
Imagine a scenario where an AI news bot, tasked with reporting on a developing crisis, hallucinates details. It might fabricate quotes from officials, exaggerate the severity, or even invent casualties. This fabricated information could spread like wildfire on social media, causing panic and hindering real emergency response efforts.
2. Healthcare Risks: When AI Makes a Misdiagnosis
The stakes are even higher in healthcare. AI-powered systems are increasingly used to analyze medical scans and assist with diagnoses. However, an AI model hallucinating a tumor where there’s none could lead to unnecessary and potentially harmful procedures. Conversely, overlooking a real health issue due to an AI hallucination could have devastating consequences.
3. Supply Chain Nightmare: Hackers Exploit the Mirage
Software development often relies on tools that recommend code libraries. Here’s where AI hallucinations can become a hacker’s playground. Imagine an AI tool hallucinates the existence of a useful software package. Hackers could exploit this by creating a malicious package with the same name, hoping developers download it unknowingly. This could introduce vulnerabilities into countless software applications, creating a nightmare for software supply chains.
Why AI Hallucinates
Now that we’ve explored the dangers of AI hallucinations, let’s lift the hood and examine the inner workings that can cause them. Here are two key factors:
1. Input Bias: Garbage In, Garbage Out
Imagine feeding a talented artist nothing but pictures of cats. Their paintings, while impressive, might struggle to depict a realistic cow. Similarly, AI models are only as good as the data they’re trained on. If the training data is biased or unrepresentative of the real world, the AI will develop skewed perceptions. This can lead to hallucinations when encountering new information that falls outside its limited understanding.
For example, an AI trained on news articles might learn to associate certain ethnicities with crime. This bias could lead to hallucinations when analyzing social media posts, potentially misinterpreting neutral language as criminal intent.
2. Adversarial Attacks: When Malice Meets Machine Learning
AI models are susceptible to manipulation by malicious actors. These “adversarial attacks” involve feeding the AI carefully crafted inputs designed to trigger hallucinations. Imagine showing a self-driving car a manipulated stop sign that looks real to humans but confuses the AI’s image recognition.
Adversarial attacks pose a serious threat to AI security, especially in applications like facial recognition or spam filtering.
How RAG Can Combat AI Hallucinations
Now that we’ve unveiled the dangers and causes of AI hallucinations, it’s time to explore solutions. Enter RAG, a powerful framework that acts as a beacon in this navigational challenge. RAG stands for Retrieve, Analyze, Generate, and each step plays a crucial role in combating hallucinations:
1. Retrieve: Building a Foundation of Truth
Remember the biased artist struggling with cows? RAG tackles this by emphasizing diverse and unbiased training data. Imagine the artist now having access to a vast library of images encompassing the animal kingdom. This diverse data provides a stronger foundation for the AI to learn from, reducing the likelihood of biased hallucinations.
2. Analyze: Demystifying the Mirage
RAG goes beyond simply feeding good data. It also incorporates analysis to identify patterns of hallucination. Imagine a detective meticulously examining crime scenes to understand a criminal’s MO. Similarly, RAG analyzes AI outputs to understand the root causes of hallucinations. This knowledge is crucial for developing targeted mitigation strategies.
3. Generate: Refining the Response
Finally, RAG leverages the insights from analysis to fine-tune the AI model. Think of the artist now receiving feedback on their cow paintings, gradually improving their ability to depict different animals. In the context of AI, RAG refines the model’s generation process to minimize the production of nonsensical or inaccurate outputs.
Practical Steps to Mitigate AI Hallucinations
Having explored the dangers and solutions through the lens of RAG, let’s delve into some practical steps we can take to mitigate AI hallucinations:
1. Building a Strong Foundation: Robust Training Data
Remember the importance of diverse data for RAG’s “Retrieve” step? This translates into a real-world action: curating high-quality training datasets. We need data that reflects the real-world’s richness and complexity, encompassing various viewpoints and experiences. By minimizing bias in training data, we significantly reduce the chances of biased hallucinations cropping up later.
2. The Human Touch: Oversight with a Critical Eye
AI is powerful, but it’s not infallible. Human oversight remains crucial in catching AI hallucinations before they cause problems. This means regularly reviewing AI-generated outputs with a critical eye, identifying nonsensical or inaccurate information. Imagine a fact-checker meticulously combing through an article – that’s the kind of scrutiny AI outputs might require in certain contexts.
3. Learning from Experience: Adaptive Algorithms
The best way to get better is to learn from your mistakes. This applies to AI as well. We need to develop models that are adaptive, meaning they can adjust their behavior based on new information and feedback. Imagine a student constantly refining their understanding based on a teacher’s corrections. Similarly, adaptive AI models can learn from past hallucinations and become less susceptible to them in the future.
AI hallucinations are both intriguing and perilous. As we embrace the power of AI, let’s also wield it responsibly, safeguarding our digital realms from mirages that threaten our security.
Remember, just as we discern shapes in clouds, our AI systems sometimes see things that aren’t there. It’s time to bring clarity to the fog of hallucination and secure our digital future.
At Maagsoft Inc, we are your trusted partner in the ever-evolving realms of cybersecurity, AI innovation, and cloud engineering. Our mission is to empower individuals and organizations with cutting-edge services, training, and AI-driven solutions. Contact us at contact@maagsoft.com to embark on a journey towards fortified digital resilience and technological excellence.